Discoveries
How to Conduct Sex Differences Research: A CREWHS Panel Preview
Mar 01, 2023 Cassie Tomlin
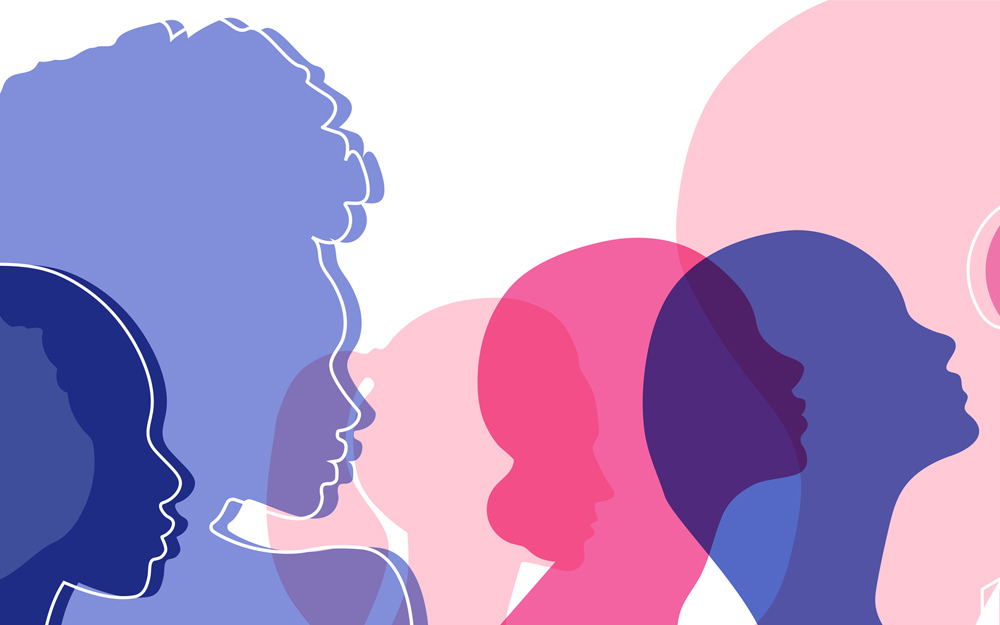
After centuries of exclusion and decades of effort to balance the scales, women are now represented almost as much as men in federally funded clinical trials.
In 2015, the National Institutes of Health (NIH) required that studies not only include women and female animals and cells in medical research, but that researchers consider sex as a biological variable in design, analysis and reporting.
The rule, and how investigators can successfully follow it, are the topic of an upcoming panel discussion to be held at Cedars-Sinai’s Center for Research in Women’s Health and Sex Differences (CREWHS) annual symposium March 14.
In advance of the event, Discoveries spoke with two panelists, Graciela Gonzalez-Hernandez, PhD, vice chair for Research and Education in the Department of Computational Biomedicine, and research veterinarian Sandra Duarte, DVM, executive director of Comparative Medicine.
Dr. Gonzalez-Hernandez’s research leverages natural language processing in AI algorithms to better glean meaning from large text sets like electronic health records, medical literature and social media. In 2022, she and colleagues published a paper, in the Annual Review of Biomedical Data Science, on best practices in using big data to identify sex bias in research.
Dr. Duarte advises investigators on which animal models are most suitable for their studies and reviews study protocols to ensure that investigators follow the NIH mandate.
There are explanations behind all of these decisions not to study females. But the result is the same: You end up with biased observations, because you start with a biased study. Good science is sometimes inconvenient."
– Dr. Graciela Gonzalez-Hernandez
What led the NIH to require that studies consider sex as a biological variable?
Sandra Duarte: Females have complex physiology, so the most common explanation has been that it is logistically too challenging to include them in the studies. Female hormonal fluctuation requires more data points—so instead of dealing with those variables, some studies exclude the female population entirely.
Sometimes investigators only want to use individuals of one sex when studying a certain disease because the other sex with the condition may die sooner. They want animals they can use from start to finish so they can collect everything they have planned—instead of asking why one of the groups is dying sooner.
Graciela Gonzalez-Hernandez: For a time, women were also excluded from research because of the risk that they could become pregnant and the study might inadvertently damage the pregnancy. There are explanations behind all of these decisions not to study females. But the result is the same: You end up with biased observations because you start with a biased study. Good science is sometimes inconvenient.
How can researchers successfully include sex as a biological variable?
GGH: It’s very important to start with something as simple as balancing the biological sexes in a study cohort to match the population, which as we know is almost 50/50.
You cannot make observations about one group and declare those observations to be true in all other groups. Studies sometimes still refer to “subjects” as a monolith, removing any notion that there are differences among them. The NIH wants to avoid this obscuring of sex as a biological variable by asking researchers to address it.
Is the aim of inclusion always to uncover differences?
SD: Yes, and also to open the mind to possibilities outside of what a researcher initially intends to study. It’s an opportunity to expand curiosity.
GGH: Sometimes, a well-designed study might find no differences between the sexes. It could turn out that men and women react the same way to disease, or a treatment has the same effect in males and females. But this must be observed—one cannot just assume.
How can research that analyzes existing data compensate for potential gaps in gender inclusivity?
GGH: Let’s say you’re working with data that’s collected already, and you’re analyzing surveys whose respondents are 75% male and 25% female. There are many solutions to make up for that disparity: You could reduce the male population to equal the population of the females, or you could issue new surveys to women to make up the difference with a complementary data set.
The important thing is that when the data that you’re using is already imbalanced, you address it in a well-reasoned, clearly stated way, either in analysis or by adjusting the source data. You can’t just say "This is an existing data set and we cannot do anything about it." That’s not good enough.
In the blog: Graduate Students Are Using AI in Medical Imaging
What are the lesser known consequences for research that doesn’t carefully consider sex as a biological variable?
SD: If at the end of the day your data is not conclusive because you were missing sex diversity, you’ve wasted so many resources. Trying to save in the short term results in more expense, and more time in the long term to go back and get it right.