Discoveries
Artificial Intelligence at Cedars-Sinai
May 27, 2022 Cedars-Sinai Staff
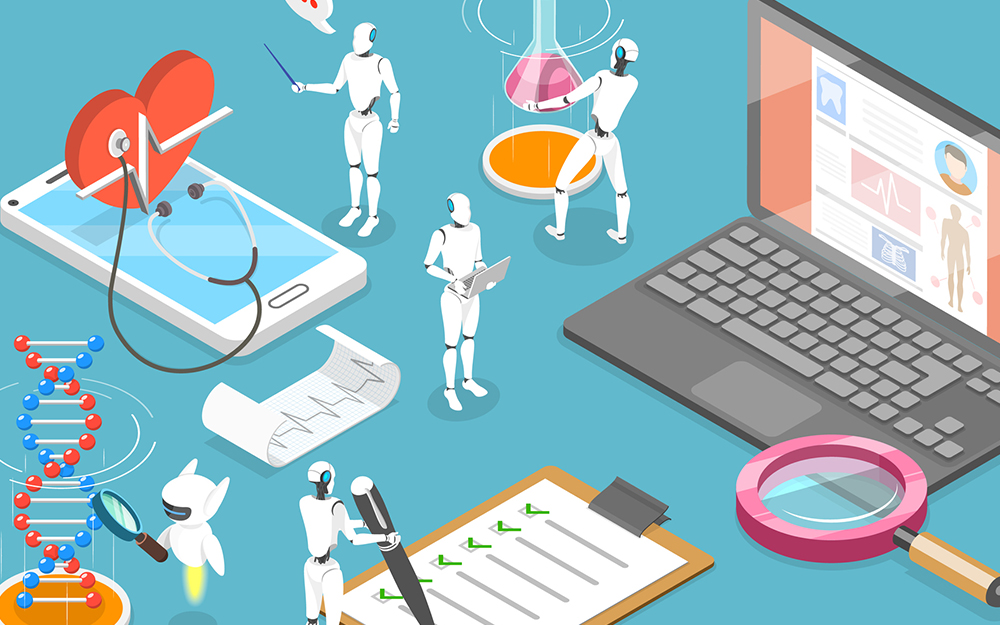
Cedars-Sinai clinicians are harnessing advanced computer technologies to expand crucial knowledge about the most devastating medical problems. Artificial intelligence (AI) is no substitute for expert clinical judgment, but investigators are leveraging machine learning to help glean insights into disease prediction and assist with speedy detection.
Scientists seeking to better treat patients with stroke, heart disease and cancer are building algorithms that make sense of numerous tiny data points. Cedars-Sinai’s new Division of Artificial Intelligence in Medicine (AIM) works in partnership with the health system's clinical data warehouse, which maintains secure health information for more than 6 million patients.
AIM experts are training AI algorithms to decode connections in vast amounts of data, and measuring their impact on patient care, says Dr. Sumeet Chugh, director of the division and associate director of the Smidt Heart Institute. Eventually, they hope to develop other aspects of AI, including discovery of novel drugs or existing medications that can be repurposed to treat new conditions, robotic surgery and computer vision.
"Eventually, the reach of AI can extend all the way from diagnosis to prediction to treatment," Dr. Chugh says. "There's a lot of genetic data connected to disease, and we can train AI to do deep learning to connect disease with genes and proteins, ultimately leading us to better tests and novel biologic treatments."
Here, an overview of AI research at Cedars-Sinai, within the Division of AIM and beyond.
Eventually, AI can really extend from diagnosis, to prediction, to treatment."
– Sumeet Chugh, MD
Heart
Researchers are using AI to sort through nuclear and CT scans to predict heart attack risk, analyze echocardiograms to better detect rare heart conditions, and distinguish between risk of fatal and treatable sudden cardiac arrest.
Sudden cardiac arrest occurs when an electrical impulse goes awry and the heart suddenly stops beating, halting blood flow to the brain and other vital organs. The condition is fatal in 90% of cases and, until recently, has been difficult to predict.
Dr. Chugh and his team developed an algorithm that accurately predicts which patients will develop treatable forms of the condition. The findings may eventually help clinicians identify patients at high risk and prevent the condition more effectively.
"We see an opportunity to predict treatable cases of sudden cardiac arrest and nip them in the bud," Dr. Chugh says. "We might have a chance of saving more lives."
He says he’ll continue to hone the novel prediction algorithm and eventually evaluate it in clinical trials for potential use in routine clinical care.
Stroke
Radiologists in the S. Mark Taper Foundation Imaging Center have developed AI to make detection of life-threatening stroke and other illness quicker and more accurate. The software processes all CT scans to detect pulmonary embolism, cervical spine fracture and subtle brain bleeds, among other critical conditions, swiftly flagging the most dangerous and time-sensitive cases.
Dr. Marcel Maya, co-chair of the Imaging Department, is studying whether clinical use of AI can shorten the length of inpatient stays. Preliminary results show that, since the Imaging Department began using AI, hospital stays for patients with brain bleeds have been reduced an average of 1.7 days.
"We’re studying whether this trend is related to earlier detection of critical findings, which could be leading to quicker diagnosis and treatment," Dr. Maya says. "That, in turn, could be preventing complications and getting patients home faster."
Pancreatic cancer
Investigators across Cedars-Sinai have collaborated to develop AI software that predicts, based on CT scans, a patient's risk of developing pancreatic cancer—which could potentially enable physicians to diagnose the devastating disease earlier.
Dr. Debiao Li, director of the Biomedical Imaging Research Institute, trained the program to compare CT scans of patients with confirmed pancreatic cancer to scans taken within six months to three years prior to their diagnosis—and also to scans of healthy patients. In a pilot study, the model could identify which patients would eventually be diagnosed with pancreatic cancer.
"Even though the scans present nothing clinically significant that can be discerned by radiologists, there is hidden information within them that could predict future cancer," Dr. Li says. "We don’t know if the cancer is already there and just too small to detect by the radiologist's naked eye, or if there are early tissue changes that precede the cancer development—but we know something is different in the CT scans."
Dr. Li, co-principal investigator of the project, and Dr. Stephen Pandol, director of Basic and Translational Pancreas Research, along with their AI team, will continue to refine the model. They will analyze additional scans of patients from seven other medical centers taken up to 10 years prior to diagnosis.
"The hope is that we can detect cancer early, and keep following patients," Dr. Pandol says. "We could closely monitor them to catch the cancer early when surgery can still be performed to remove the cancer and save more people's lives."
AI DEFINED
Scientists at Cedars-Sinai are training cutting-edge computer technology to assist with research. Here, a few definitions:
Artificial intelligence (AI)
Any tool in which computers are trained to perform functions that usually require human thought, such as "reading" a CT scan
Machine learning
A tool used to develop AI; a technique in which computers are trained to analyze and learn data and then improve their own knowledge or accuracy, such as learning to recognize patterns in CT scans
Deep learning
A category of machine learning; a technique in which a computer trains itself to perform a task and make intelligent decisions, such as identifying specific diagnoses in CT scans