Discoveries
Reducing Bias in AI Models
Apr 20, 2023 Cedars-Sinai Staff. Photograph by Al Cuizon.
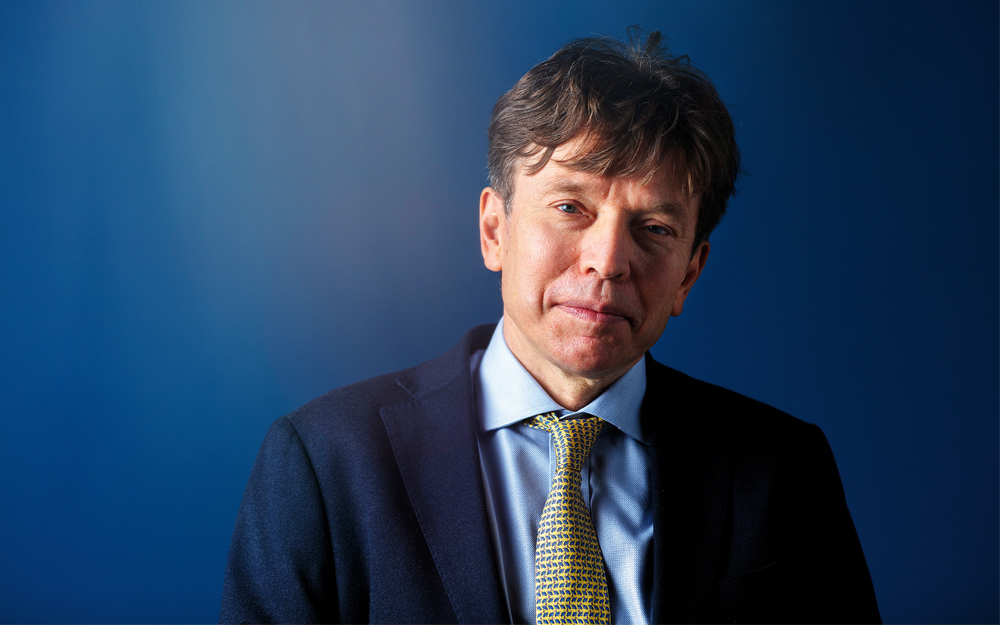
AI systems are most robust when they are built on broad data sets drawn from a diverse array of patients. A Cedars-Sinai team published a study in the European Journal of Nuclear Medicine and Molecular Imaging that describes how to train an AI system to perform well in all applicable populations—not just the specific population the system was built on.
Some AI systems are trained using high-risk patients, which can cause overestimation of disease probability. To ensure that the AI model works accurately for all patients and to reduce bias, Piotr Slomka, PhD, and his team trained their AI system using simulated variations of patients to scan images to predict heart disease.
The team found that models trained with a balanced mix of cases more accurately predicted the probability of coronary artery disease in women and low-risk patients, which can potentially lead to less invasive testing and more accurate diagnosis.
The models also led to fewer false positives, suggesting that the system may reduce the number of tests the patient undergoes to rule out the disease.
"The results suggest that enhancing training data is critical to ensuring that AI predictions more closely reflect the population that they will be applied to in the future," says Dr. Slomka, director of Innovation in Imaging at Cedars-Sinai and a research scientist in the Division of Artificial Intelligence in Medicine and the Smidt Heart Institute.
Read more about the promise of integrating AI into medicine in our special report: