Discoveries
Living in AI’s Endless Summer
Apr 20, 2023 Nicole Levine. Illustration by Kirsten Ulve.
It’s not enough for algorithms to be accurate. They must be trustworthy, explainable and unbiased.
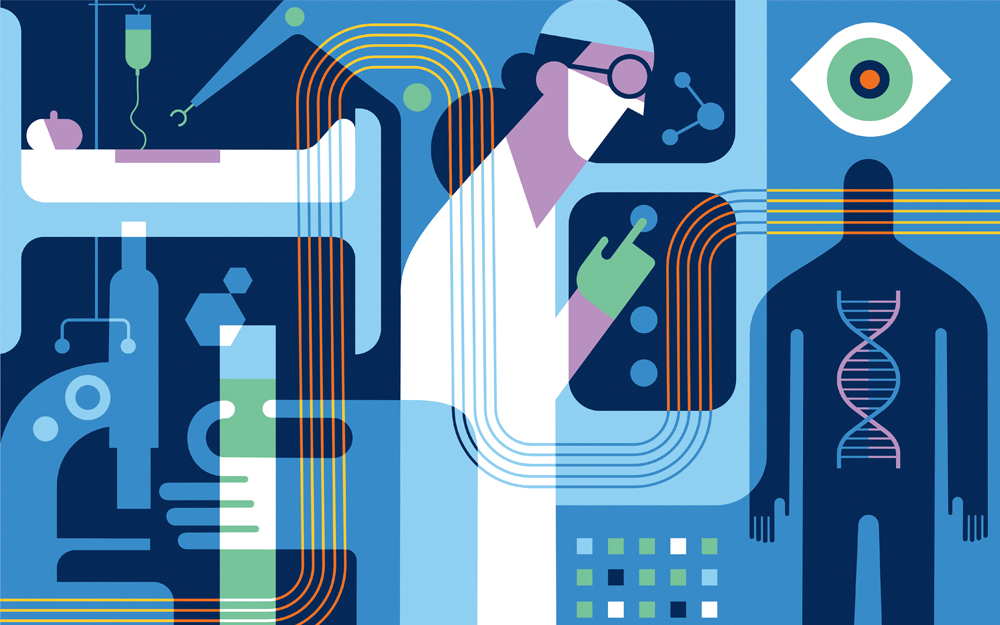
Artificial intelligence helps schedule hospital staff, assign beds, guide robotic surgery, and analyze genetic and imaging tests—while reshaping scientific discovery. Since the 1950s, when an artificial intelligence-driven computer successfully played a game of checkers, AI has hibernated through winters of little progress and boomed through scientific summers of advances. Now, the endless summer of AI has arrived—for healthcare as well as virtually every other industry. Poised at a crossroads where AI could push care in a direction that is patient-driven—or could perhaps generate distrust—it’s the human factor that matters most.
"In this endless summer, the question is how can we harness the power of AI so that it can eventually make the care of our patients better, more accurate and more efficient?" says Sumeet Chugh, MD, director of Cedars-Sinai’s Division of Artificial Intelligence in Medicine. "To answer that question, we need doctors and researchers dedicated to keeping patients at the center of AI development. So much AI has come from big technology and has not been conceived and born in a health system."
AI has powerful potential to assist scientists in making groundbreaking medical breakthroughs and free up doctors to spend more time focusing on patients. To unlock that potential requires partnerships that span medical and technological disciplines, and a commitment to leverage only unbiased, comprehensive data. Health systems must invest resources to move to the front lines of these innovations—to help shape the tools of healing and discovery, Dr. Chugh says.
Creating an AI algorithm with the potential for deep learning—wherein it continues to learn and evolve as it digests more data—is complex. Using one is not. From a technical standpoint, such tools are ready to proliferate to the average clinical computer workstation as readily as similar technologies have permeated our smartphones.
"We already have the technology to make AI accessible to anybody who wants it," says Jason Moore, PhD, chair of Computational Biomedicine at Cedars-Sinai. Dr. Moore has spent close to a decade developing tools to make AI accessible and effective for clinicians. "The real challenge is creating trustworthy models that are sound enough to assist in making clinical decisions."
The Democratization of AI
Dr. Moore and his team have already created software that acts as an easy-to-use data science assistant (see sidebar). It’s a snap to learn, and Dr. Moore notes that similar tools are also available commercially.
"The struggles with putting AI into clinical practice are not technological," Dr. Moore says. "It’s the broader questions—like how to make sure you’re addressing the bias in the data and that the AI is giving high-quality, clean results."
Dr. Chugh’s division focuses on deploying AI tools in research and care that are calibrated to patient needs. His group includes clinicians, researchers and machine-learning engineers.
"Because we’re clinicians and scientists who are embedded in healthcare, we can identify where we have gaps in medical knowledge. We use large amounts of data—which are ethically vetted and secured with appropriate safeguards—to drill down on very specific questions," says Dr. Chugh, who is also medical director of the Smidt Heart Institute’s Heart Rhythm Center and holds the Pauline and Harold Price Chair in Cardiac Electrophysiology Research. Enhancing diagnostic assessments, improving imaging and predicting sudden cardiac arrest are among the important AI interventions Dr. Chugh’s team is developing.
"It’s time to challenge some assumptions and dogma about how we predict and prevent disease, because without doing a more effective job of matching treatments to those who will benefit from them, our healthcare model isn’t sustainable," Dr. Chugh says. "It’s up to the medical community to drive that. Health systems have an opportunity to pose the right questions and develop AI that keeps patients at the center."
Read more about the promise of integrating AI into medicine in our special report:
The Black Box Problem
The one question AI is not very good at answering is a crucial one: Why?
AI has a black box problem, says Dr. Moore. We know the questions being posed and the data fed to the algorithm. We know the predictions and answers the AI tool generates. But in a deep-learning scenario, it can be impossible to determine how it arrived at its answers.
"A clinician must always be able to ask why, and the answer needs to be understood clearly by the providers and by their patients," Dr. Moore says. "Right now, that’s a major limitation of modern AI. It’s not good at the ‘why’ question."
Explainability requires extra effort by an AI tool’s creators to track the algorithm as it iterates and to be able to present the path it took to arrive at an answer.
"If the algorithm is so complex that even the developer cannot understand how it works, it’s not going to be a good candidate for use in healthcare," Dr. Moore says. "That’s why our teams develop transparent AI systems that encourage the sound and ethical use of the technology."
In this same critical moment when AI is filtering into wider use, clinicians and scientists are locked in a quest to achieve health equity. Scientists recognize and are addressing the significant role discrimination based on race, sex, sexuality, gender, poverty and other factors plays in health outcome disparities. An algorithm based on data with inherent bias could amplify and magnify those disparities.
"We must always keep the clinician in mind as we develop and evaluate AI tools," Dr. Moore says. "We need to think first about what’s good for all patients and how we earn the trust of those charged with their health and wellbeing. Our teams are dedicated to capturing the benefits of innovation and applying them equitably."
AI Integration
Building AI that will seamlessly blend into the complex workflows of a busy medical center, laboratory or physician’s office and improve the patient experience is key to its adoption, says Michael Thompson, vice president of Enterprise Data Intelligence. That means building programs that span medical and academic disciplines, administration, and technology departments.
"Often, artificial intelligence stops at publishing a paper," Thompson says. "Many programs are created in universities rather than a hospital setting, causing a massive gap between the mathematical AI models and the people and systems that need to use them."
One AI tool spanning research and logistical functions at Cedars-Sinai is ALEx, named for "automated learning by example." ALEx is a helpful colleague, developed to assist in research and fulfill functions that free up nurses and doctors to spend more time with patients. That ALEx was created by and for medical professionals made a difference, Thompson says.
"As we help move AI into a clinical workflow and into a patient setting, our goal always is to make sure that it can add value to patient outcomes. We make sure that the clinician who is using the results of AI knows how to use it appropriately," he says. "We created a comprehensive strategy and approach to using AI across the board—in patient treatment, in research, in testing and in hospital operations and with our values driving that strategy."
Managing the human element of AI is essential to deploying it effectively and applying it equitably in healthcare.
"We believe in innovation, in discovery and in moving the needle so the patient benefits," Dr. Chugh says. "AI is a tool that can do that if—and only if—we keep the core questions we’re asking of it close to the patient."
Accessible AI
Data Science Assistants for All
AI tools are part of everyday operations at Cedars-Sinai, assisting in the lab and the clinic so their human counterparts can work more effectively.
Aliro
Imagine if every clinician had a data science assistant. With Aliro, they can, says Dr. Moore. Aliro allows clinicians and researchers with no machine-learning or coding expertise to run analysis through a sleek web interface. Anyone who can find their way around a spreadsheet or load a data set into a web browser can master this tool.
Aliro learns from experience and remembers every analysis it’s ever completed, allowing it to improve over time.
ALEx
ALEx is a powerful AI tool aiding Cedars-Sinai professionals in capacities that span many disciplines and departments—from helping with the logistics of placing patients to assisting with research and even finding savings on surgical supplies.
Matching resources to ever-fluctuating demands is a daily challenge for medical centers, and ALEx has proved a reliable partner for several years. The AI assistant is used daily in the Capacity Command Center to synthesize thousands of forecasts into useful patterns to aid in determining staffing needs, placing patients, discharge planning and other patient flow operations.
During the COVID-19 pandemic, ALEx used public health data to assist with bed planning, staffing, service-line planning and supply chain needs. ALEx also helped generate models to assist in the transition when the medical center reopened for elective surgeries.
ALEx has a role in research, such as predicting which patients are likely to require C-section deliveries.