Discoveries
An Objective Approach to Facial Feminization Surgery
Jun 29, 2023 Sarah Spivack LaRosa
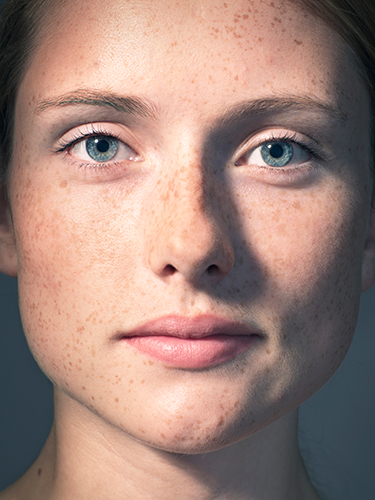
Human faces exhibit stunning diversity, and we immediately draw conclusions about other people based on the shape and size of their faces.
Gender is one of the attributes we assign after the merest glance at a person’s face. Facial feminization surgery, which changes the shape of the face to look more feminine, can achieve gratifying results for people experiencing gender dysphoria, a feeling of discomfort or unease that arises when the sex a person was assigned at birth does not match their gender identity.
The tricky part for plastic surgeons who specialize in facial feminization is determining exactly how to alter a patient’s existing facial characteristics. Our perception of genders is based on several components—such as age, race and ethnicity—and it is difficult to objectively determine what makes a face appear feminine.
Reconstructive plastic surgeon Edward Ray, MD, and a multidisciplinary team that includes Jason Moore, PhD, chair of the Cedars-Sinai Department of Computational Biomedicine, and his scientist colleague Xiuzhen Huang, PhD, have developed a machine-learning algorithm to help quantify exactly what moves surgeons should make to ensure a face will be perceived as female to a casual observer.
Facial feminization surgery includes a broad array of procedures, such as forehead and hairline contouring, jaw and chin reduction, and rhinoplasty.
To help establish feminization guidelines, Ray’s research group gathered anonymous information from patients who underwent CT scans. The investigators fed the data, including the gender and age of each patient, to their algorithm to give the machine a baseline of features that qualify as male or female.
They then provided the algorithm with pre- and post-operative scans of patients who had undergone facial feminization surgery to determine if the system could differentiate the images by gender appearance. Surgeons worked with the scientists who trained the algorithm to help interpret what areas of the face are most indicative of masculine or feminine features.
“Now, for an individual surgery, the algorithm provides a heat map-like mesh of a patient’s face with a colored gradient that can guide surgeons to focus on certain areas: to set back the redder areas and pull out the bluer ones, for example,” Ray said. “The mesh is tailored to each patient, so it has the potential to be a very powerful tool.”
The best way to create the mesh is to provide the algorithm with hundreds of image files, each representing one slice of a face. A surgeon can tell the tool that it is looking at a 27-year-old Latino male, for example, which will further define how to feminize the skull. “But even without accounting for such specifics, we have reached 85%-95% accuracy working with a few hundred CT scans,” Ray said.
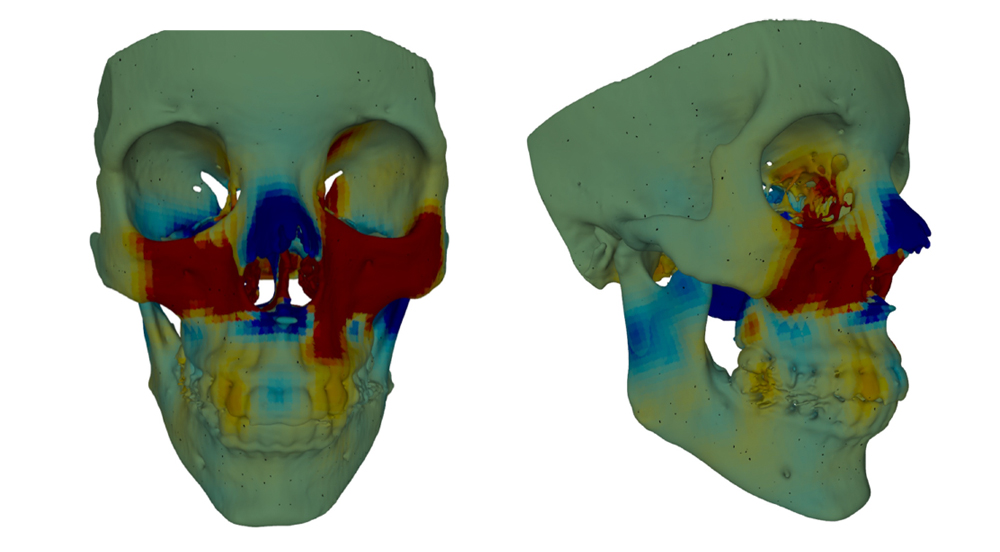
A machine-learning tool generates this heat map-like mesh of a patient's face that guides surgeons to focus on certain areas during facial feminization procedures.
After a surgeon has completed an operation with the assistance of this artificial intelligence tool, the team will ask the surgeon how helpful it was. They will also examine facial scans after surgery to determine if the patient, in fact, achieved a more feminine look, using the algorithm to grade the procedure’s success. Patients will report on how satisfied they are with the results.
“The eye picks up on minute details when determining gender. This tool is trying to get us over the threshold to ensure post-operative patients will appear how they wish to appear,” said Victor Chien, MD, director of Cleft and Craniofacial Surgery at Cedars-Sinai and a collaborator on the research.
Ray is still developing the machine-learning tool and plans to feed thousands more data points to the algorithm to hone its recommendations.
“It is important to emphasize that every decision made during a surgical procedure is based on that surgeon’s judgment. Eventually, we may be able to say, ‘Go ahead and trust the algorithm to make the decisions,’ but the technology is like a self-driving car, and we are not going to take our hands off the steering wheel yet,” Ray said.
This multidisciplinary group has conducted a small proof-of-concept study and is pursuing a larger, multisite investigation. Ray noted that the research may have application in lymphedema reconstruction, where the lymphatic system has been damaged because lymph nodes were removed due to cancer. This can occur after breast cancer surgery.
“The standard of care is to look at scans that map the flow of dyes into the lymphatic system. If we can collect a large number of those images, we may be able to use machine learning to prognosticate which patients are going to benefit from which operation,” he said.