Research Areas
Specific projects in the lab span various computational approaches and include:
Faster Methods for Proteomic and Metabolomic Data Collection
We are innovating new ways to collect proteomic and metabolomic data more quickly and completely. We recently described a method to enable fast proteomic analysis by direct infusion shotgun proteome analysis (DISPA) (Meyer, et al. Nat Methods. 2020). We subsequently published how this method can be used for fast, targeted quantification of specific proteins. (Trujillo, et al. Anal Chem. 2022.)
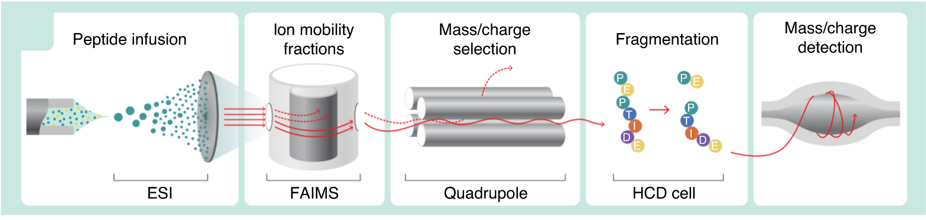
Proteome Informatics
We develop tools to enable analysis of new types of data, and tools to extract more knowledge from existing proteomics data. We developed a tool that uses peptide correlation analysis (PeCorA) to infer protein post-translational modifications in bottom-up proteomics datasets. We showed how this can reveal direct evidence of PTMs, indirect evidence of PTMs, and poorly quantified peptides that should be excluded from analysis.
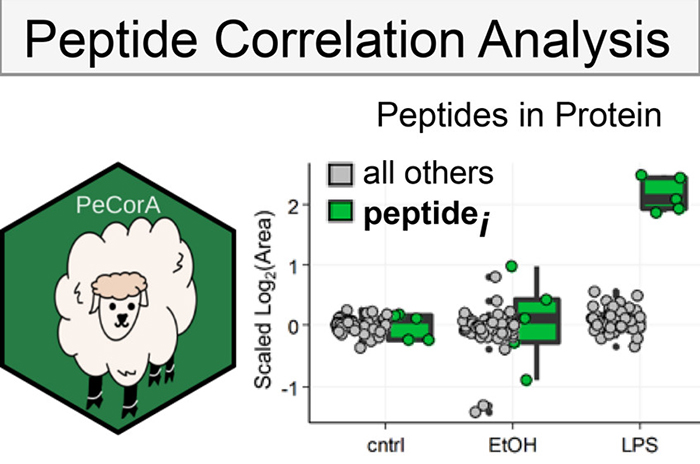
We also described a software tool for analysis of the unique data we generate with DISPA that we hope will enable other labs to try this method (Cranney, Meyer. Anal Chem. 2021). In making this tool we found several ways to make the data analysis faster and more sensitive, including a pooled spectra scoring scheme and a new scoring function.
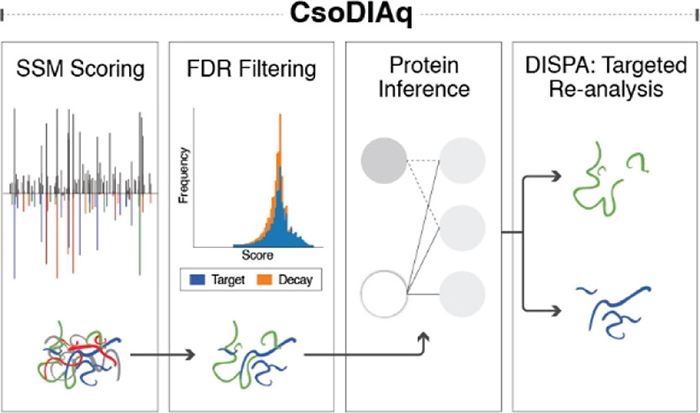
Multi-omic Data Integration
Multiple layers of omics data should provide more insight than the sum or the parts. We are building new methods to integrate across omic layers that provide new biological insights. One recent method we described is multi-omic data integration by machine learning (MIMaL), which can reveal new connections between two omic layers—e.g., between proteins and metabolites. (Dickinson, et al. bioRxiv. 2022.)
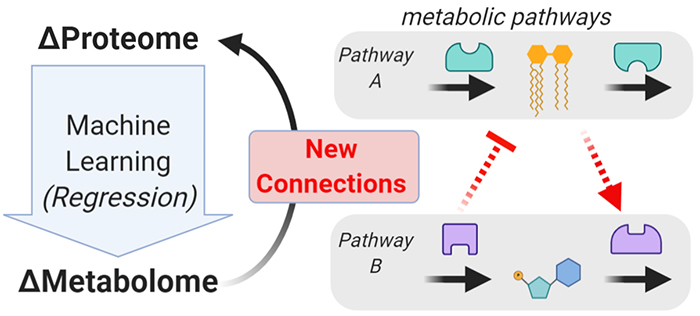
Applied Interpretable Machine Learning
We apply machine learning to understand electronic health records (EHR) and other biological data. We have described how different types of EHRs contribute to clinical predictions, and how model interpretation can reveal biases in the model and data. (Momenzadeh, Shamsa, Meyer. medRxiv. 2022.)
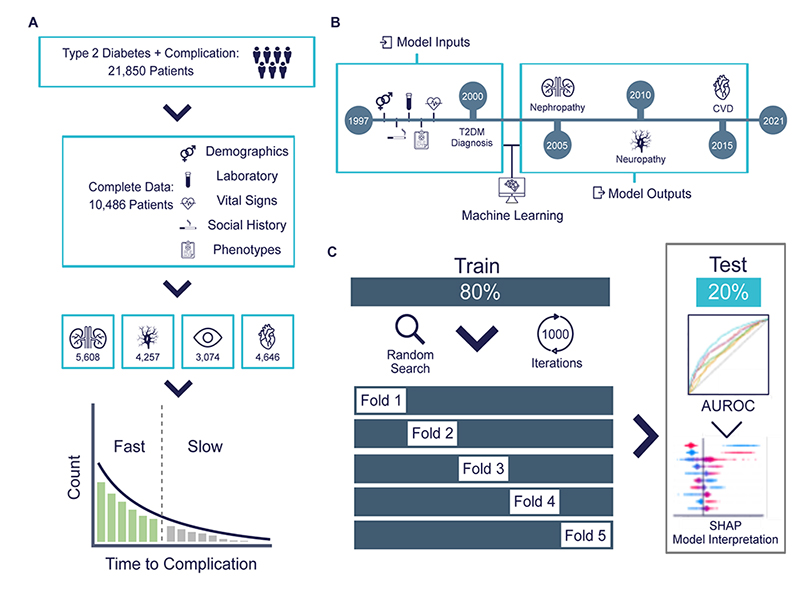
We also use model interpretation methods to interpret models trained to predict properties of biological sequences. We wrote a tutorial on how model interpretation can reveal linear motifs that regulate peptide binding to different major histocompatibility complex alleles in humans and simians, or interactions between amino acids that determine peptide collisional cross sections in ion mobility mass spectrometry. (Dickinson, Meyer. PLoS Comput Biol. 2022.)
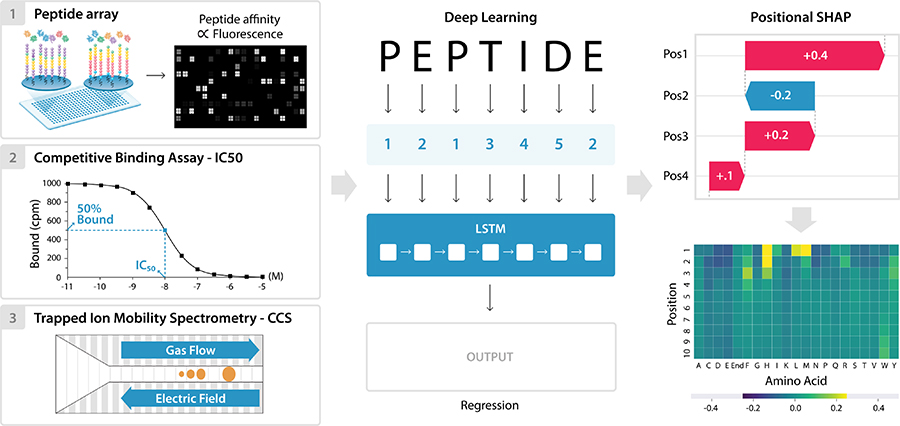
We have also used machine learning to predict general chemical effects and showed that even with less than 1000 training examples, transfer learning enables convolutional neural networks to approach baseline performances from random forest models. (Meyer, et al. J Chem Inf Model. 2019.)
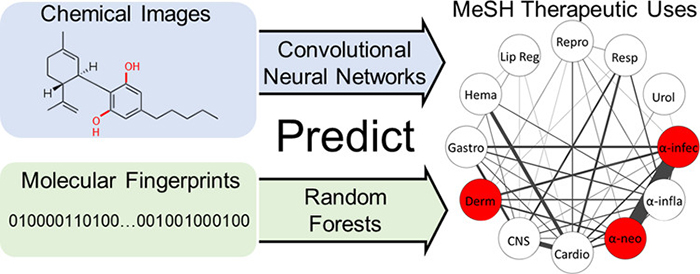
Drug Discovery for Alzheimer’s Disease
We are developing new drug discovery paradigms using iPSC-derived neurons, artificial intelligence and high- throughput omics. We have received preliminary funding from an NIH R21 and are growing this project now.